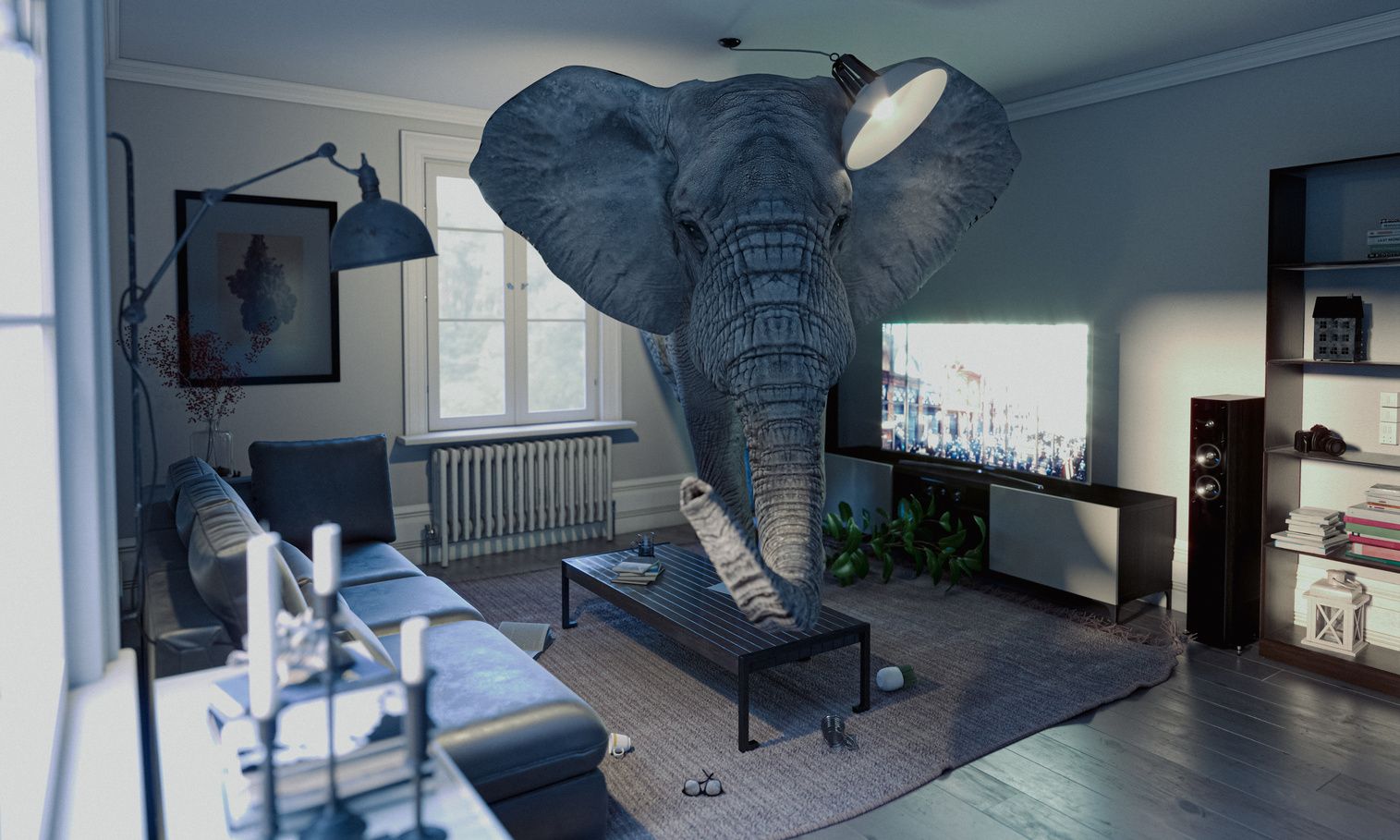
Why are our video ads underperforming?
At Lineate we often hear this question from publishers and SSPs. That’s why we’ve significantly improved video ad performance by applying optimization techniques around integration and configuration, and by using predictive modeling to introduce dynamic floor pricing and request filtering.
Many techniques for optimizing programmatic advertising apply to video, but video can intensify traffic-quality problems. Since the rest of the ad ecosystem is moving away from cookies, the solutions to video will become more and more applicable to the industry as a whole.
Defining the problem . . . and the solution
We measure video ad performance by the rate of bids received against available video inventory and the price of these bids. These two metrics are at odds because increasing the acceptable-pricing threshold drives down the bid rate — this is the problem.
To find a solution we must first define success: maximizing the bid rate with a minimal price threshold, maximizing the pricing with a minimal bid or fill rate, or maximizing overall revenue from both the price threshold and the bid rate.
The next step: iterating through hypotheses to find effective changes. Hypotheses rely on data; fortunately, adtech industry players are sitting on a ton of data. So our analysis begins with selecting the worst-performing units (publishers or ad placements) and filtering them by priority (revenue impact) and the probability of finding something. After testing our ideas we broadly introduce the best results.
Filtering out low bids with predictive modeling
Many publishers don’t set floor prices for video ads, and the result is devalued inventory, low revenue, and difficulty winning upstream auctions. Our remedy is to introduce default floor prices and dynamic floor pricing optimized to the defined success criteria.
Some publisher agreements preclude floor pricing, which poses a problem unique to video ads. The publishers receive low-quality bids of little value, but the required OpenRTB responses are costly. Our predictive model enables us to nix the low bidders’ requests in advance, allowing the publisher to devote resources to more promising traffic.
In addition, demand for video ads can be artificially reduced by configuration issues, such as when, in the OpenRTB stream, certain fields are missing or set incorrectly. Problems also occur when parameters correlate with poor quality, or when publishers have an integration issue.
When a publisher’s winning bids lose in upstream auctions it signals a traffic-quality problem. We can’t measure this result, but we can find it by comparing auction-win rates against tracked impressions and click numbers; larger discrepancies may indicate a problem. We’ve introduced this signal into the dynamic floor-pricing algorithm, enabling publishers to suppress poor traffic and boost their impressions and win rate.
Cookieless: The elephant in the room
Unmatched traffic without cookies is the main source of traffic-quality problems and dramatically affects bid activity, especially for video ads, which are prominent in apps that have non-cookied traffic by default. We resolve this issue with a first-party data exchange to ensure we’re sending fundamental demographic data such as IAB categories in the RTB request. We can’t directly fix unmatched traffic without cookies, but we can figure out what publishers need to do to fix the problem. However, because video is less likely to have cookies traffic, video ads are very adaptable to upcoming changes in the ad tech ecosystem.
Share:
Got a project?
Harness the power of your data with the help of our tailored data-centric expertise.
Contact us